AIOps stands for Artificial Intelligence for IT operations. It means using machine learning and big data to analyze data streams. It works well with anything from process logs to the way tickets are handled in a data center or analyzing the revenue. The results translate to increased process efficiency, augmented productivity, and, ultimately, more profit.
How to use AIOPs to boost KPIs
Tom Peters said: “What gets measured gets done.” Robin Sharma added: “What gets measured gets improved.” They are both right, and most companies keep a close eye on their KPIs to be sure that they are doing the right tasks and that these tasks are being improved whenever possible. In this scenario, AIOps can be a beneficial tool to help decision-makers and change for the better the way the organization operates.
Identify problems fast and accurately
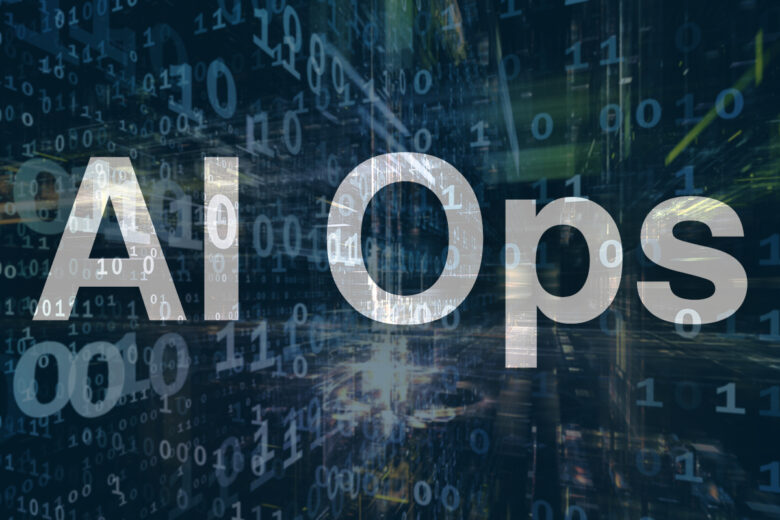
Data-structure agnostic
Only analyzing historical data during the monthly or quarterly reviews is outdated. AIOps can identify issues almost in real-time, sometimes within seconds, by putting together insights from various data sources: structured tables, semi-structured data like XML files, and non-structured such as customer feedback. The sooner a problem is signaled, it can be fixed, preventing downtime and other losses. This feature is vital for those managing servers, live broadcasting, and other services that require continuity. Any failure in these areas could trigger clients to switch to competitors.
Properly trained machine learning algorithms can pinpoint problems on the spot, thus boosting KPIs almost instantly.
The immediate applications include process KPIs. The best candidates for these measurements are those KPIs which measure progress and keeping on track:
- Cost variance (CV) (planned budget vs. actual budget)
- Schedule variance (SV)
- Schedule performance index (SPI)
- Cost performance index (CPI)
- Planned hours of work vs. actual situation
These could be kept through a simple spreadsheet if you have a small, in-house team, but in the globalized environment of today, automated and aggregated tools powered by AI give you a competitive advantage.
Anomaly detection
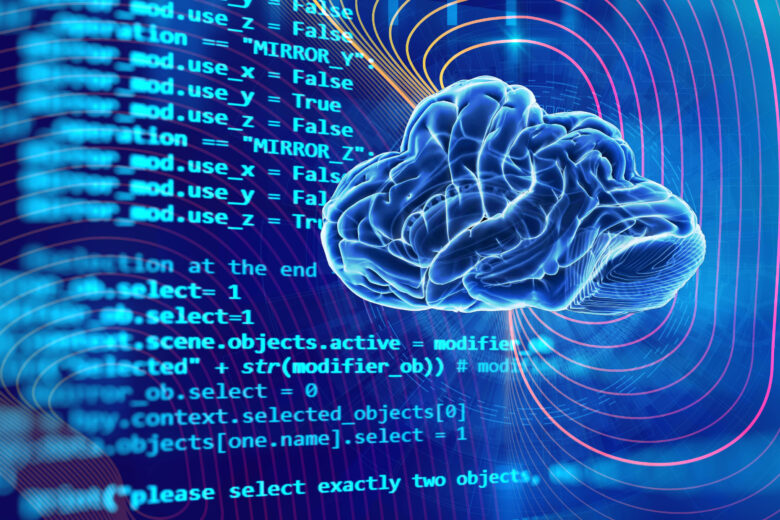
For example, in anomaly detection, they learn the right patterns and identify any deviation from what is considered normal. Using AIOps in this way helps companies provide uninterrupted service. It can help banks prevent frauds and improve the business’s quality, positioning the organization as responsive and responsible. AIOps can have a wide range of different applications, including noise reduction, root cause analysis, prediction, and more.
Any issues of the underlying IT infrastructure usually translate to substantial business losses. AIOps can notably influence the KPIs related to service quality, user experience, uptime, and customer retention rates.
These tools can also integrate seamlessly with different platforms, including ticket management and customer service. Once the problem is signaled, the automated processes notify the right teams by assigning tasks. This is a way to speed up the solution and to get more work done.
Trend Analysis and Trend Estimation for Security and Productivity
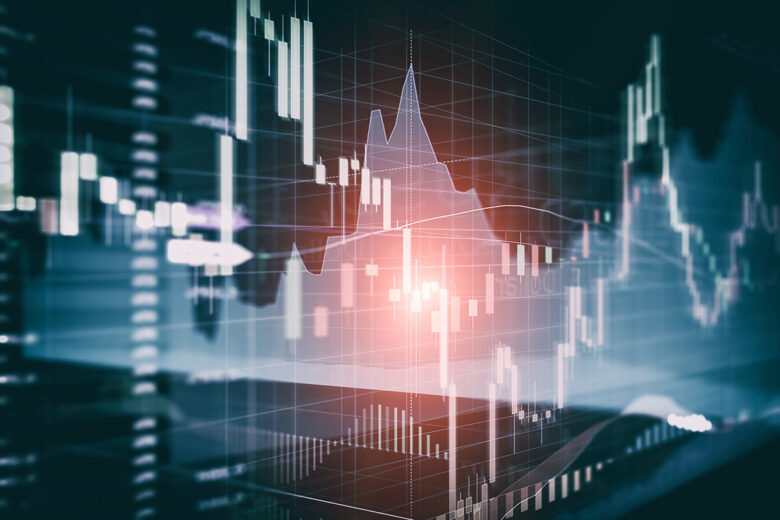
AIOps dashboards can signal unnatural occurrences in trends that are then quickly analyzed by IT staff members and correlated with real-life events such as outages.
Looking at historical data and comparing it with actual recordings can help improve KPIs like:
- Accuracy of estimates
- The average time to repair,
- Average handle time.
When it comes to the delicate problem of data security, pattern detection can signal frauds about to happen by noticing unusual activity on the network.
Resource Optimization
Adopting AIOps in your organization means less time spent on firefighting issues and more time for added value work. Automating issue detection and having real-time warnings means streamlining the processes.
When it comes to KPIs, these improvements could increase the number of:
- Projects delivered on budget by decreasing overtime spent fixing issues
- Critical bugs fixed
- Tickets solved promptly.
Potential drawbacks of using AIOps
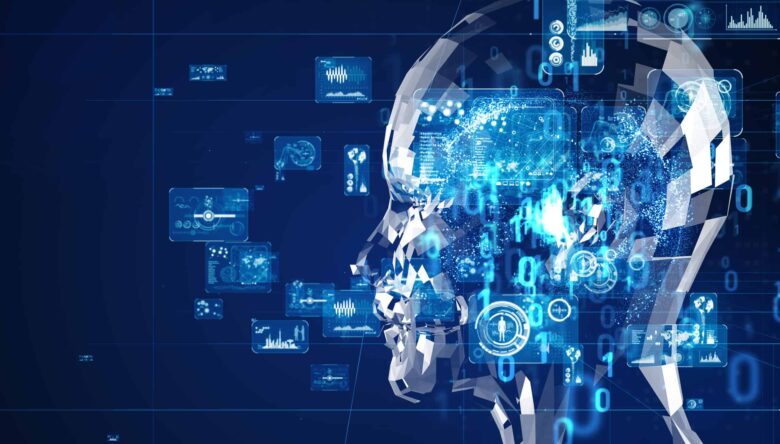
Although this is a powerful tool, it has numerous limitations and requires a specific environment to function correctly. Any company interested in the technology should know that there is no one size fits all, and top results require customization to the company’s processes. In most instances, these processes need to go under a re-evaluation before using AIOps.
The quality and quantity of data
For an AIOps solution to work, it needs large amounts of data to train the underlying algorithm and function properly. If your company is a start-up and you do not have enough data to use as a reference, this might not be the best choice, even if it is the latest technological hype.
Data quality is also paramount since incomplete, inconsistent, or missing data can’t be used to create a robust system.
For example, if you are trying to implement AIOps in your organization’s ticketing department, you need appropriately categorized data. Suppose your current records don’t have assigned categories, urgency, and problems. In that case, it can be challenging to create a prediction of the most common ticket issues and allocate staff members to solve them.
High inertia rates for new technology adoption
Mr. Henry Ford had a point with his observation about the “faster horse”. Most people want an improvement in their current work environment without making fundamental changes to the tools they are used to and how they work.
However, they have unrealistic expectations from the results they should expect when implementing AI solutions in their organizations. They fail to collect, clean and tag data; they are not willing to calibrate the tools and often under-utilize powerful and costly solutions, only to become frustrated and blame the vendors.
Check if AIOps is right for you
Although AIOps can have numerous benefits, it is a high-end solution that requires adaptation to a new way of working and should be introduced gradually in an organization.
It is most suitable for companies that operate with data streams that verify the 3 Vs. of Big Data: volume, velocity, variety (according to AIOps Deployment | Siscale).
The quick wins revolve around less downtime and streamlining operations. Simultaneously, the advantages, in the long run, imply reliability and essential cost reductions.