The importance of data science has been increasingly stressed with the rise of technological analysis and data gathering. However, many companies are still getting their footing on proper applications of data science and analytics—hire a team of analysts, or leave it up to software? In reality, there are a variety of departments in which data science could boost performance.
Gathering insights and essential statistics from the data available to a business is a crucial consideration for making informed decisions and moving the company forward effectively. Big Data is meaningless without the proper software and experts in place to turn it into a reliable tool. Harnessing the power of data to turn numbers into insights can vastly improve business processes, from human resources to logistics.
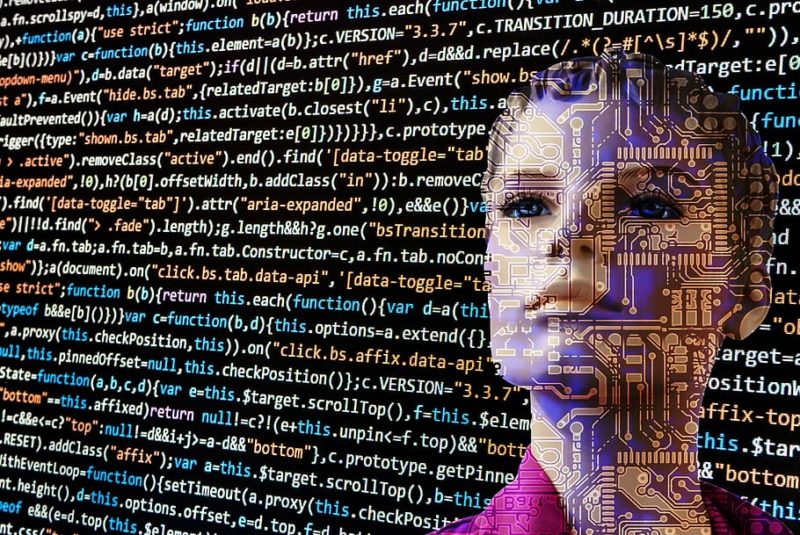
1. Large-Scale Analysis
Big Data needs to be put through a series of algorithms and processes to provide any meaningful information, and this is where large-scale data analysis comes in. Its importance reflected both in academics and business. The purpose of the large-scale study is to provide a consistent flow of actionable information from a large dataset.
The process of sorting through and storing data for analysis is often accompanied by distributed file systems (DFS). This effectively unifies the data across a company’s processes into a manageable, accessible, and organized operation. Often, data scientists can take it from here, gathering any unusual patterns and statistics to extract any insights from the data sets. However, a DFS is often accompanied by analysis software on the same scale, which can provide graphs, correlations, or even insights of its own. You can click for more here if you want to explore it thoroughly.
Whatever the implementation of large-scale analysis, it’s vital to do this effectively. Vast sums of data provide nothing unless they are appropriately stored and meaningfully analyzed by the respective software and/or experts. In that sense, data science plays a massive role in turning gigabytes into valuable decisions.
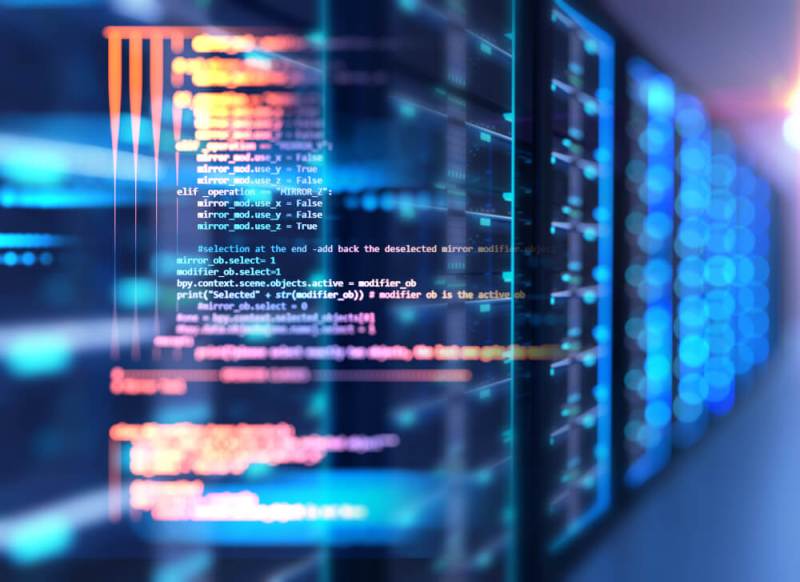
2. Predictive Analytics
Predictive analytics is especially crucial for retailers today, as it can vastly improve pricing strategies if implemented correctly. Specifically, having a foothold on price optimization, elasticity, and simulations can make or break a business, especially in the retail sphere. Of course, none of these are particularly simple to crack, which is why data science plays a huge role.
Anything from competition to branding strategy can impact price optimization, so having a large-scale data analysis system in place is essential for knowing the “right” prices to set. At its core, price optimization software and processes seek to answer two questions: how will customers react to certain pricing decisions, and what prices best reflect our company objectives? Today, with the ability buyers have to compare prices, price optimization is a complex task that is often undertaken by machine learning and AI-based tools.
Price elasticity, while a bit more straightforward, still requires a fair bit of forethought. Price elasticity of demand, in short, is used to reflect the relationship between changes in a product’s price and changes in demand for it. For example, if demand for a product falls drastically with a price increase, the product is very elastic—implying that there are enough substitutes on the market for it. Of course, many non-pricing factors affect price elasticity of demand, such as brand recognition or loyalty. The purpose of data science, then, is to identify these factors (as outlined here) and how to work around the best.
Pricing simulations can also offer some insight into how different prices can affect sales, demand, engagement, etc. This can be as simple as running necessary calculations for impact on sales, or complex enough to account for a variety of market factors. Effective use of price simulations with data science can act as a feedback loop of sorts, depending on the complexity of software in place. If you’re interested in data science and improve your data fluency, you may check out QuantHub.
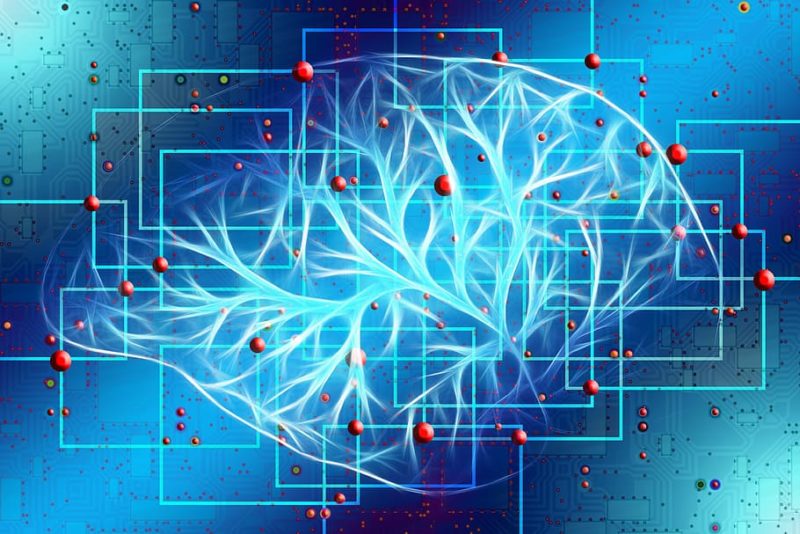
3. Decision-Making in Business
In the end, data science should be a tool that improves decision-making across the board. Proper business analytics helps managers understand the dynamics of their company, anticipate impacts of market changes, and mitigate any risks accordingly. Planning changes and approaching business processes with a forward-thinking mindset brings consistency and stability to a business.
Businesses that use data analytics to impact their decision-making often find success in operational efficiency and customer satisfaction. Using the power of data to shift business processes to a more scientific approach allows businesses to edge out competitors using traditional methods in customer acquisition, loyalty, and profitability.
In short, data science can vastly systematize and optimize a business’s operations across the board. Effective data analysis offers insights and patterns that can help both understand market effects on business and mitigate any risks involved with specific decisions.
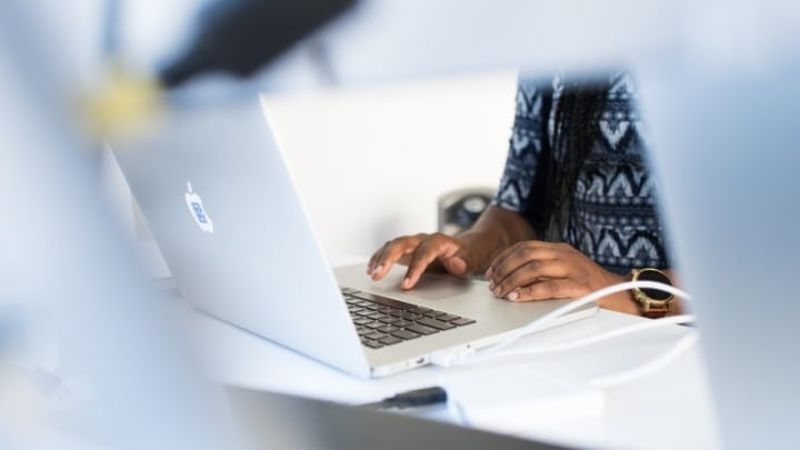
4. Data Science Methods in Human Resource Management
Even in the realm of HR, data science, and emerging technologies like artificial intelligence can improve and overhaul relevant processes. Taking an analytical approach to finding, hiring, and retaining employees can make HR processes more straightforward and resource-efficient.
Data science is useful for collecting and analyzing information about a candidate’s qualifications and skills, the bulk of which is aggregated from social media, forums, and similar online activity. Reliable algorithms can then gather insights from this seemingly-irrelevant data to evaluate a candidate’s professionalism or overall personality.
Similarly, data science can be implemented in workforce analysis and estimation of required investment. Data-driven software can return forecasts, estimates, and suggestions based on a variety of factors, from the position to the candidate/employee’s preferences. By setting these tools in line with the company’s objectives and needs, data science can be a welcome instrument in expediting and optimizing HR processes.
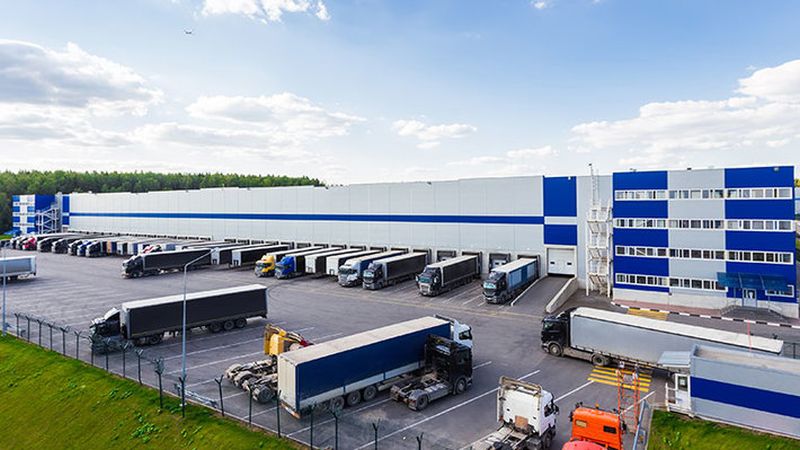
5. Data Science in Logistics and Transportation
Of course, data science goes hand-in-hand with logistics processes, be it inventory management or truck routing. Using data to monitor logistical processes is vital for warehouse optimization, demand forecasting, sufficient throughput rates, reducing freight costs, and identification of breakdown patterns (to name a few).
Fluid Logistics and transportation processes are built on efficiency, and data science brings just that. By getting a transparent view of every detail within a process chain, businesses can more easily identify areas to tweak or overhaul with the patterns and insights that data-focused tools can bring. The important thing, as with any application of data science, is to have a clear-cut approach to storing, organizing, and analyzing relevant data to improve both small and large-scale decisions.